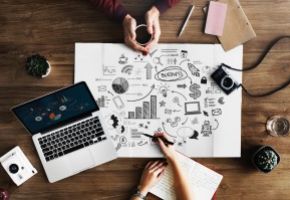
iSmentors: How to predict the popularity of video content in social media using artificial intelligence?
Popularity prediction of online content has gained a lot of attention within the research community due to the ubiquity of the Internet and a stunning increase in the number of its users.
Popularity prediction of online content has gained a lot of attention within the research community due to the ubiquity of the Internet and a stunning increase in the number of its users. Multiple factors render the popularity prediction of social media content a challenging task, which includes propagation patterns, a social graph of users and the interestingness of content. Solving this problem has multiple applications for modern social media video creators and publishers. An accurate prediction of a video’s popularity gives helpful feedback while creating a video on a given topic and helps in the choice of appropriate promotion.
Current methods for online content popularity analysis focus mostly on determining its future popularity. Although popularity prediction is an important issue , we believe that it does not address all the challenges faced by online video creators. More precisely, predicting popularity does answer the question many creators have: how a given frame or title word contributes to the popularity of the video. Even though correlation does not mean causality, this kind of analysis allows for the understanding of the importance of a given piece of content and, accordingly, for the prioritizing of the creation efforts.
We have outlined a fundamentally different approach to online video popularity analysis that allows social media creators to both predict video popularity and to understand the impact of its headline or video frames on future popularity. To that end, we have proposed using a self-attention based model and gradient-weighted class activation maps, inspired by the recent successes of the attention mechanism in other domains.
METHOD
Since our work is focused on predicting the popularity of video content in social media, we collected data from Facebook, the social network with the highest number of users, with a reported 1.18 billion active daily users worldwide. To collect a dataset of videos, along with their corresponding view counts, we implemented a set of crawlers that use Facebook Graph API and ran it on the Facebook pages of over 160 video creators listed on the social media statistics website TubularLabs. To avoid crawling videos whose view counts are still changing, we restricted our crawlers to collecting data on videos that had been online for at least 2 weeks. Besides the videos and their view counts, we also collected both the first and the preferred thumbnails of the videos, as well as the number of the publishers’ followers. Any Facebook videos without any of this information were discounted. The resulting dataset consists of 37 042 videos published between 1st June 2016 and 31st September 2016. It is worth noting that Facebook increments the viewcount of a video after no less than three seconds viewing time and, by default, turns on the autoplay mode. Therefore, in our experiments, we focused on the beginning of the video and take as input of the evaluated methods representative frames from the first six seconds of a video.
To reduce any bias stemming from the fact that popular publishers receive more views on their videos, irrespective of their quality, we divided the number of views of a video by the number of followers of a publishers’ page and used this value as a normalized view count.
We cast the problem of video popularity prediction as a binary classification task to predict whether the normalized view count of a video would be below or above the median value.
IMAGE MODALITY
To predict the popularity of a video, we used 18 frames uniformly extracted from the first 6 seconds of a video. We use the deep convolutional neural network ResNet50, pre-trained on the ImageNet dataset, to get a high-level image descriptor for each frame. To obtain a compact vector representation, we trained a two-layer neural network with attention on top of the extracted features. Thanks to this learnable attention mechanism, the model was able to learn which frames from the sequence contribute most to popularity prediction.
TEXT MODALITY
Videos posted on social media are usually posted with a short text description that can be thought of as a variable length sequence of words. We explored the use of this description in video popularity prediction. We used pre-trained GloVe vectors to represent text as a sequence of meaningful word vectors and trained a bi-directional LSTM network with attention to predict popularity class. Attention mechanism allows for a learning focus on words that contribute more to the popularity score.
MULTIMODAL APPROACH
Image and text may contain complementary information about video content. We combined the two domains to improve the results of popularity prediction. To that end, we concatenated two vectors: a video embedding and a headline embedding from separately trained models and trained a two-layer neural network for popularity prediction on top of that.
RESULTS
We evaluated the performance of our methods using classification accuracy, computed simply as a ratio of correctly classified samples on the hold-out test set. As a complementary performance metric, we used Spearman correlation between video probabilities of belonging to a popular class and their normalized popularity score. This is because one of the goals of the proposed model is to help the creators to make a data-driven decision on which video should be published, based on its predicted popularity.
Method | Accuracy [%] | Spearman correlation |
Video frames | 68.87 | 0.526 |
Headline | 68.70 | 0.525 |
Multimodal | 72.72 | 0.607 |
The results of different modalities
Interestingly, almost equal popularity prediction results can be obtained using either video frames or headline features. Combining both modalities leads to a noticeable improvement of up to 17% for Spearman coefficient and 4 p.p. for accuracy.
VISUALIZATION
A strong advantage of our method is that it can be used to visualize parts of image and text that contribute to predicted popularity score, which can help understand popularity patterns found by our model. In the image domain, we use Grad-CAM to create heatmaps that highlight parts of frames relevant to the prediction of popular class. To weight the influence of each frame in time domain, we scaled the heatmaps by weightings obtained from the attention mechanism.
In the text domain we use weightings obtained from the attention mechanism directly for visualization.
The visualizations provided by our method may offer insights into what influences a video’s popularity and can be used for image thumbnail selection or headline optimization.
Selected video frames with their highest and lowest predicted popularity scores
Videos related to food with warm colors tend to get higher scores. Videos related to news, with with poor exposition, or with cold colors tend to get lower scores.
CONCLUSIONS
Our work shows new approaches for popularity prediction of social media videos using a self-attention mechanism in video frames, textual and multimodal domain. We have showed how using both visual and textual features allows for more accurate predictions. More importantly, we have proposed a method that combines Grad-CAM with a soft attention mechanism to visualize which parts of a video contribute to its popularity in both the spatial and temporal domains. Along with using attention to visualize the influence of words in video description, we have showed an entire system that increases interpretability of popularity prediction methods for social media videos. Future research includes more fine-grained visualizations using attention on convolutional feature maps, learning coordinated modality representations, headline generation based on a video.
Tags:
See also:
LATEST NEWS
Infoshare 2025 | Shape Tomorrow Today 10.02.2025
Ticket sales have started! | Infoshare 2025 23.01.2025
Infoshare Startup Contest 2025 is... ON! 🔥 09.01.2025
Dziękujemy za sezon 2024! 31.12.2024
Warsztat Infoshare Katowice: Zamień observability w przewagę 20.11.2024
Zapisz się na Round Tables, Speed Dating i Matchmaking! 🗣 13.11.2024